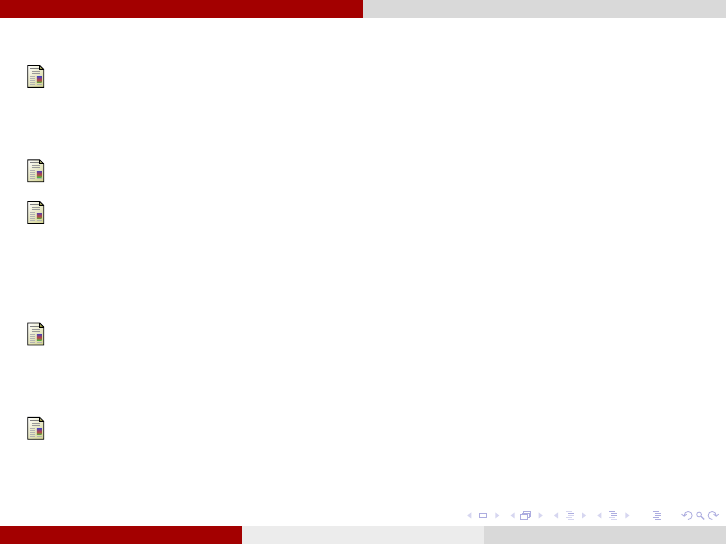
References
[12]. Fares Qeadan & Brianna Killian (May, 2007). BtB: An Analysis
of a Cognitive Behavioral Therapy Technique used in Treating
Depression. Final project for STAT 775 (Dr. DoHwan Park).
[13] Ronald Christense (2001). Advanced Linear Modeling. Springer.
[14] Proudfoot, J., Goldberg, D., Mann, A., Everitt, B., Marks, I., &
Gray, J. A. (2003). Computerized, interactive, multimedia
cognitive-behavioural program for anxiety and depression in general
practice. Psychological medicine, 33(02), 217-227.
[15] Beck, A. T., Steer, R. A., Ball, R., & Ranieri, W. F. (1996).
Comparison of Beck Depression Inventories-IA and-II in psychiatric
outpatients. Journal of personality assessment, 67(3), 588-597.
[16] Everitt, B. S. (2006). An R and S-PLUS companion to
multivariate analysis. Springer Science & Business Media. Chicago
Fares Qeadan, Ph.D (Department of Internal Medicine Division of Epidemiology, Biostatistics, & Preventive MedicineUniversity of New Mexico Health Sciences Center)Longitudinal Data Analysis by Example April 5, 2016 29 / 31